Care work in long-term care (LTC) is considered as a genuine human-centred activity, requiring empathy, emotional investment, physical encounters and intimate, trust-based relations between various care-givers and care-recipients. AI technologies are introduced in this professional field to assist care workers in their daily activities and provide an additional measure of care for clients. This has changed the provision of care, affecting care givers and recipients alike.
So far, little research has been done on the biases that emerge from AI in this field and the risks that algorithmic governance of care offers in the profession. Based on data generated by AI technologies, unfair decisions can remain unnoticed in the process of linking different big data sets, leading to ethical and social issues in LTC.
ALGOCARE’s goal is to understand the functionality of algorithmic governing systems of care and their effects on care givers and recipients. Insight from ethnographic research in LTC will provide an understanding of the impact and needs of care in relation to AI systems. The use-value of eXplainable AI (XAI) methods (trustworthiness, privacy awareness, explainable procedures) and different levels of transparency that either the model itself provides or methods that provide them after development are explored. Based on this insight, metrics are developed to evaluate the eXplainability of machine learning models for care.
How To Address Fairness
In an algorithm, bias can be found within 3 stages. In order to mitigate bias, we can address the pre-processing step, where the training data can have underlying bias. Another stage is the in-processing stage where we look at the algorithm and its logic itself. Finally, especially if we deal with black-box models, we look at the output of the system in the so-called post-processing step in order to find post-hoc explanations of the model.
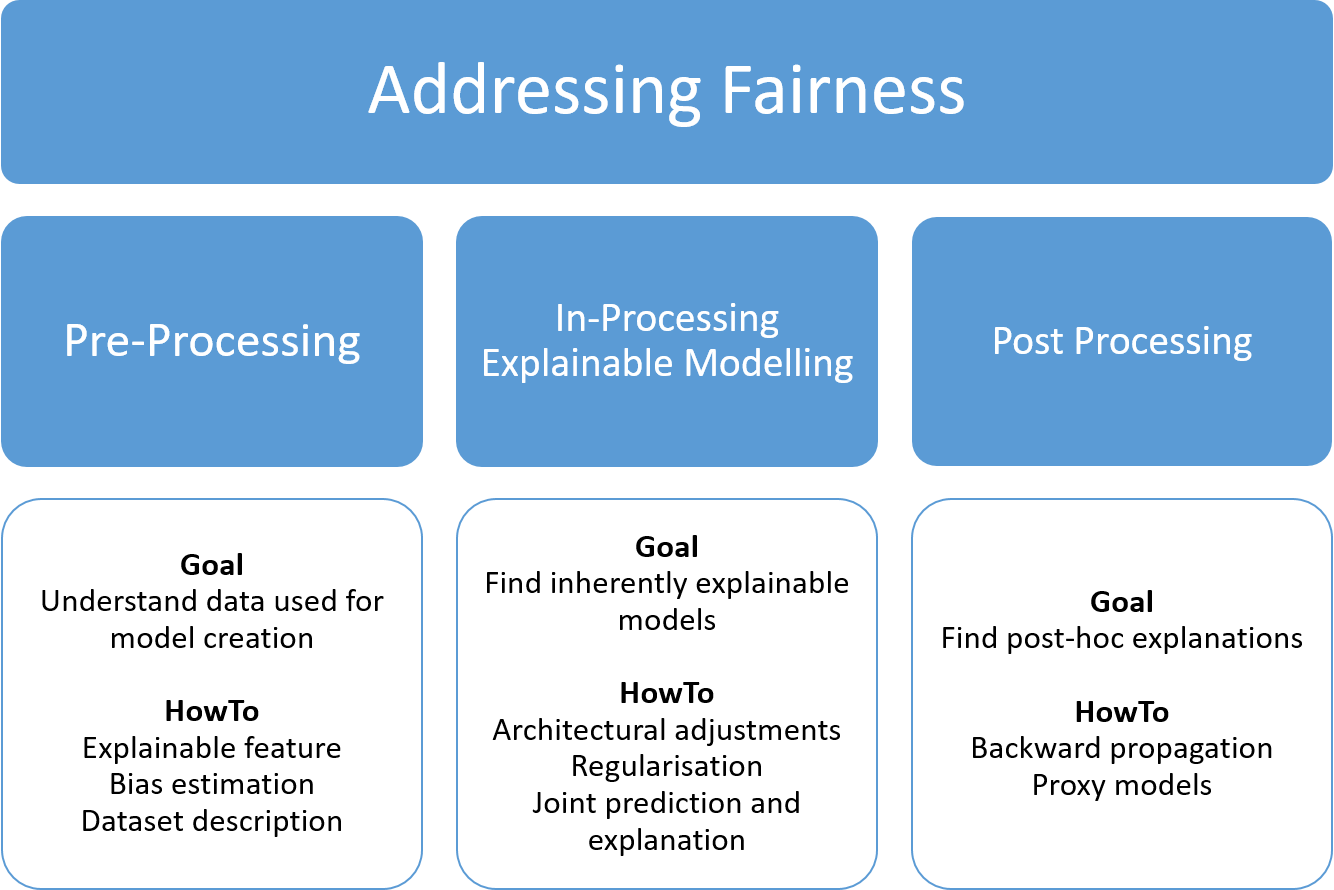
A Practical Approach to FairAI in Healthcare
What is considered a bias depends on the specific task, the legal framework and practical context. Biases are the outcome of the current state of AI that can only operate in a very narrow setting. Based on a strict empiricist functioning, outliers from the norm – omnipresent in our social reality – present major issues for current AI, as the empiric
training data is not able to cover all (potential) outliers. In addition to protected attributes that should not be used to discriminate between individuals, scalable attributes like physical disability may play a role in care. Fairness in the field of AI thus is an avoidance of various forms of bias that can be identified in data structures, calculation processes and the use of decision tools.